How Neurosymbolic AI Can Fix Generative AI’s Reliability Issues
Generative AI has made impressive strides in recent years. It can write essays, create art, and even compose music. But when it comes to getting facts right, it often falls short. It might confidently tell you that zebras live underwater or that the Eiffel Tower is in Rome. While these mistakes might seem harmless, they […] The post How Neurosymbolic AI Can Fix Generative AI’s Reliability Issues appeared first on Unite.AI.
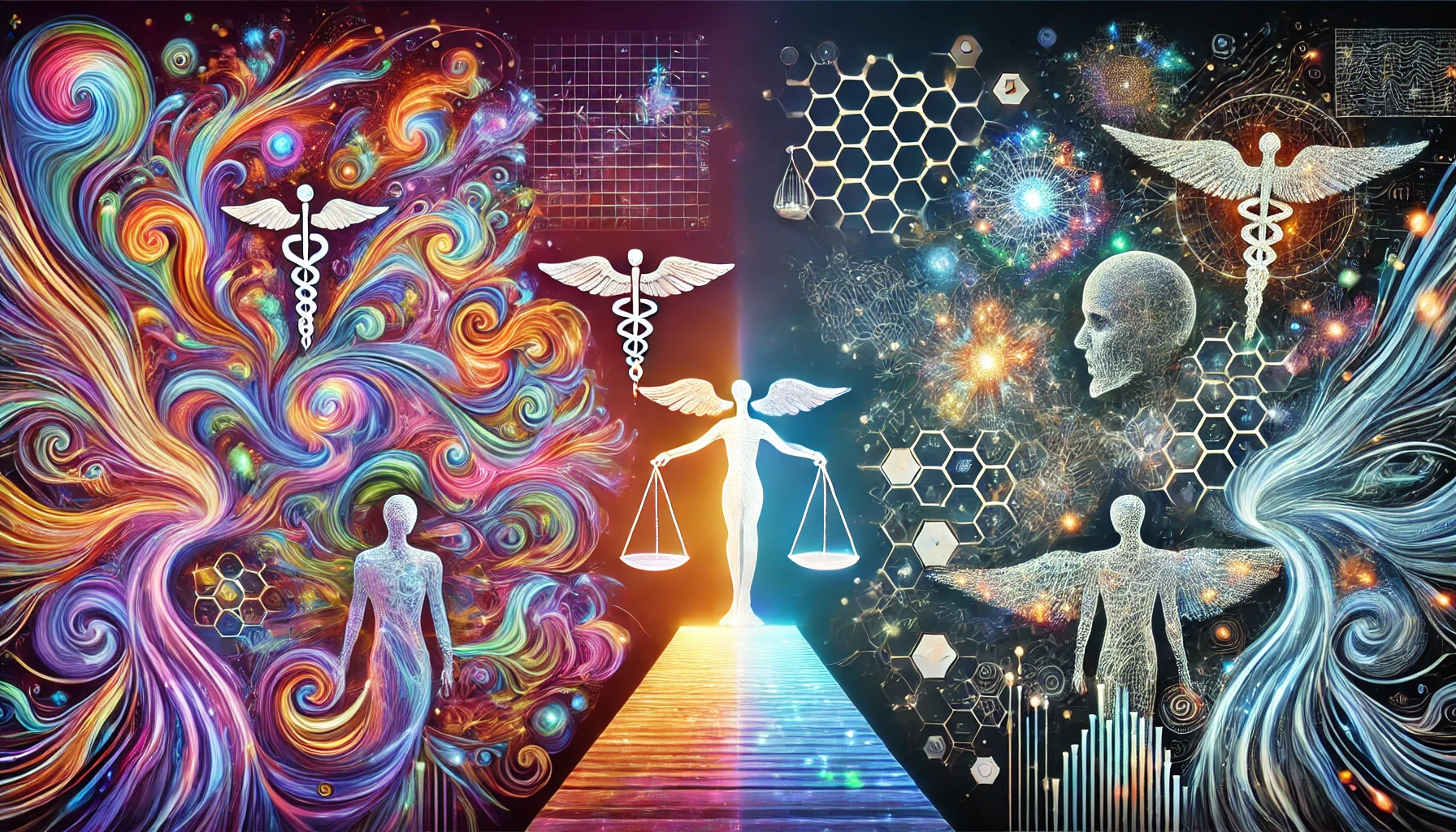

Generative AI has made impressive strides in recent years. It can write essays, create art, and even compose music. But when it comes to getting facts right, it often falls short. It might confidently tell you that zebras live underwater or that the Eiffel Tower is in Rome. While these mistakes might seem harmless, they point to a bigger issue: trust. In areas like healthcare, law, or finance, we can’t afford to have AI making such errors.
This is where neurosymbolic AI can help. By combining the power of neural networks with the logic of symbolic AI, it could solve some of the reliability problems generative AI faces. With neurosymbolic AI, we can build systems that don’t just generate answers but generate answers we can trust.
Why Generative AI is Unreliable
Generative AI works by analyzing patterns in massive amounts of data. That’s how it predicts what word or image comes next. It’s like an advanced autocomplete tool that’s incredibly versatile, but it doesn’t really “know” anything. It just plays the odds. This reliance on probabilities can make it unpredictable. Generative AI doesn’t always choose the most likely option. Instead, it selects from a range of possibilities based on patterns it has learned. This randomness can make it creative, but it also means the same input can lead to different outputs. That inconsistency becomes a problem in serious situations where we need dependable answers.
Generative AI doesn’t understand facts. It mimics patterns, which is why it sometimes makes things up and presents them as real. This tendency of AI is often known as hallucination. For instance, AI might invent a quote from a famous person or creates a citation that doesn’t exist. This is useful when we need to create new content, but can be a serious problem, especially when AI is used to give advice on medical, legal, or financial matters. It can mislead people into trusting information that’s simply not true.
To make matters worse, when AI makes mistakes, it doesn’t explain itself. There’s no way to check why it gave a certain answer or how to fix it. It is essentially a black box, hiding its reasoning in a tangle of mathematical weights and probabilities. This can be fine when you’re asking for a simple recommendation or casual help, but it’s much more concerning when AI decisions start impacting things like healthcare, jobs, or finances. If an AI suggests a treatment or makes a hiring decision, not knowing why it chose that answer makes it hard to trust.
At its core, generative AI is a pattern matcher. It doesn’t reason or think. It generates responses by mimicking data it was trained on. This makes it sound human, but it also makes it fragile. A small change in input can lead to big mistakes. The statistical foundation of AI relies on patterns and probabilities, which makes it inherently random. This can result in highly confident predictions, even when those predictions are wrong. In high-stakes fields like legal advice or medical recommendations, this unpredictability and lack of reliability pose serious risks.
How Neurosymbolic AI Enhances Reliability
Neurosymbolic AI could solve some of these reliability challenges of generative AI. It combines two strengths: neural networks that recognize patterns and symbolic AI that uses logic to reason. Neural networks are great at processing complex data, like text or images. Symbolic AI checks and organizes this information using rules. This combination can create systems that are not only smarter but also more reliable.
By using symbolic AI, we can add a layer of reasoning to generative AI, verifying generated information against trusted sources or rules. This reduces the risk of AI hallucinations. For instance, when an AI provides historical facts. Neural networks analyze the data to find patterns, while symbolic AI ensures the output is accurate and logically consistent. The same principle can also be applied in healthcare. An AI tool might use neural networks to process patient data, but symbolic AI ensures that its recommendations align with established medical guidelines. This extra step keeps results accurate and grounded.
Neurosymbolic AI can also bring transparency to generative AI. When the system reasons through data, it shows exactly how it arrived at an answer. For example, in legal or financial sectors, an AI could point to specific laws or principles it used to generate its suggestions. This transparency builds trust because users can see the logic behind the decision and feel more confident in the AI’s reliability.
It also brings consistency. By using rules to guide decisions, neurosymbolic AI ensures responses stay steady, even when inputs are similar. This is important in areas like financial planning, where consistency is crucial. The logical reasoning layer keeps the AI's output stable and based on solid principles, reducing unpredictability.
The combination of creativity with logical thinking makes neurosymbolic generative AI smarter and safer. It’s not just about generating responses—it’s about generating responses you can count on. As AI becomes more involved in healthcare, law, and other critical areas, tools like neurosymbolic AI offer a path forward. They bring the reliability and trust that truly matter when decisions have real consequences.
Case Study: GraphRAG
GraphRAG (Graph Retrieval Augmented Generation) shows how we can combine the strengths of generative AI and neurosymbolic AI. Generative AI, like large language models (LLMs), can create impressive content, but it often struggles with accuracy or logical consistency.
GraphRAG addresses this by combining knowledge graphs (a symbolic AI approach) with LLMs. Knowledge graphs organize information into nodes, making it easier to track connections between different facts. This structured approach helps the AI stay grounded in reliable data while still generating creative responses.
When you ask GraphRAG a question, it doesn’t just rely on patterns. It cross-references its answers with trusted information in the graph. This added step ensures logical and accurate responses, reducing errors or “hallucinations” common in traditional generative AI.
The Challenge of Integrating Neurosymbolic and Generative AI
However, combining neurosymbolic AI with generative AI isn't easy. These two approaches work in different ways. Neural networks are good at processing complex, unstructured data, like images or text. Symbolic AI, on the other hand, focuses on applying rules and logic. Merging these two requires a balance between creativity and accuracy, which isn’t always easy to achieve. Generative AI is all about producing new, diverse results, but symbolic AI keeps things grounded in logic. Finding a way to make both work together without compromising performance is a tricky task.
Future Directions to Follow
Looking ahead, there's a lot of potential for improving how neurosymbolic AI works with generative models. One exciting possibility is creating hybrid systems that can switch between the two methods depending on what’s needed. For tasks that require accuracy and reliability, like in healthcare or law, the system can lean more on symbolic reasoning. When creativity is needed, it can switch to generative AI. There’s also work being done to make these systems more understandable. Improving how we can track their reasoning will help build trust and confidence. As AI continues to evolve, neurosymbolic AI could make systems smarter and more dependable, ensuring they’re both creative and trustworthy.
The Bottom Line
Generative AI is powerful, but its unpredictability and lack of understanding make it unreliable for high-stakes fields like healthcare, law, and finance. Neurosymbolic AI could be the solution. By combining neural networks with symbolic logic, it adds reasoning, consistency, and transparency, reducing errors and increasing trust. This approach not only makes AI smarter but also ensures its decisions are dependable. As AI plays a bigger role in crucial areas, neurosymbolic AI offers a path forward—one where we can count on the answers AI provides, especially when lives and livelihoods are at stake.
The post How Neurosymbolic AI Can Fix Generative AI’s Reliability Issues appeared first on Unite.AI.