Machine Learning in Healthcare: Transforming Diagnosis and Treatment
In the ever-evolving and important landscape of healthcare, one technological innovation stands out as a game-changer: machine learning (ML). This subset of artificial intelligence (AI) is revolutionizing how we diagnose diseases, personalize treatments, and predict patient outcomes.
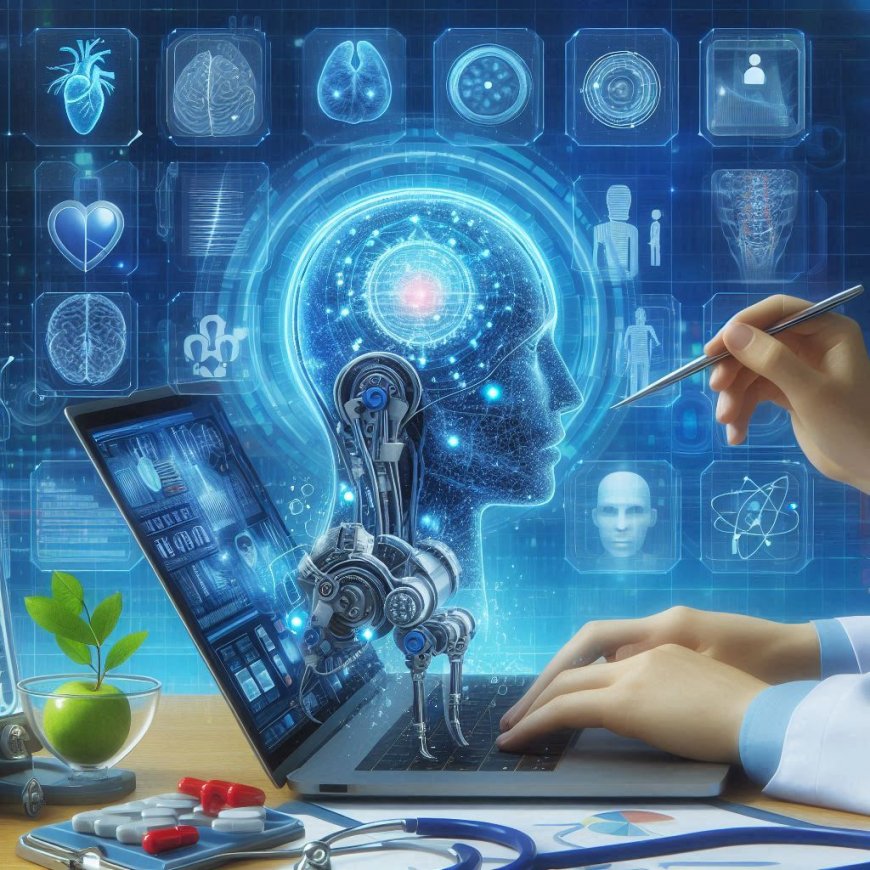
In the ever-evolving and important landscape of healthcare, one technological innovation stands out as a game-changer: machine learning (ML). This subset of artificial intelligence (AI) is revolutionizing how we diagnose diseases, personalize treatments, and predict patient outcomes. From detecting cancer in its earliest stages to tailoring drug treatments for individual patients, machine learning is not just a buzzword—it’s a transformative force reshaping the future of medicine.
The Power of Data: Fueling Machine Learning in Healthcare
At its core, machine learning thrives on data. And healthcare, with its vast repositories of patient records, imaging data, genomic information, and clinical trial results, is a treasure trove waiting to be unlocked. Machine learning algorithms can sift through this data, identifying patterns and correlations that would be impossible for humans to detect. This capability is particularly valuable in areas like diagnostics, where early and accurate detection can mean the difference between life and death.
For example, consider the field of radiology. Radiologists are tasked with interpreting complex images, such as X-rays, MRIs, and CT scans. While their expertise is unparalleled, the sheer volume of data can lead to fatigue and human error. Enter machine learning. Algorithms trained on millions of medical images can now assist radiologists by flagging abnormalities, such as tumors or fractures, with remarkable accuracy. In some cases, these algorithms have outperformed human experts, detecting subtle signs of disease that might otherwise go unnoticed.
Early Diagnosis: A Lifesaving Advantage
One of the most promising applications of machine learning in healthcare is its ability to enable early diagnosis. Diseases like cancer, Alzheimer’s, and cardiovascular conditions are far more treatable when caught in their initial stages. Machine learning models can analyze patient data—ranging from genetic markers to lifestyle factors—to identify individuals at high risk long before symptoms appear.
Take breast cancer, for instance. Researchers have developed ML algorithms that analyze mammograms to predict the likelihood of malignant tumors. These models not only improve diagnostic accuracy but also reduce the number of false positives, sparing patients unnecessary stress and invasive procedures. Similarly, in cardiology, machine learning is being used to predict heart attacks by analyzing electrocardiogram (ECG) data and other risk factors.
Personalized Medicine: Tailoring Treatments to the Individual
No two patients are exactly alike, and neither are their responses to treatment. Machine learning is paving the way for personalized medicine, where therapies are tailored to an individual’s unique genetic makeup, lifestyle, and medical history. This approach is particularly impactful in oncology, where traditional treatments like chemotherapy can have varying levels of effectiveness and side effects.
By analyzing genomic data, machine learning algorithms can identify which patients are most likely to benefit from specific drugs or therapies. For example, ML models have been used to predict how cancer patients will respond to immunotherapy, a cutting-edge treatment that harnesses the body’s immune system to fight tumors. This not only improves outcomes but also minimizes the trial-and-error approach that often accompanies cancer treatment.
Predictive Analytics: Preventing Complications Before They Occur
Another area where machine learning is making waves is predictive analytics. Hospitals and healthcare providers can and are using ML models to forecast patient outcomes, such as the likelihood of readmission, infection, or complications following surgery. These predictions enable clinicians to intervene proactively, potentially preventing adverse events and improving patient care.
For instance, in intensive care units (ICUs), machine learning algorithms can monitor patients in real time, analyzing vital signs and lab results to detect early signs of sepsis—a life-threatening condition that requires immediate treatment. By alerting healthcare providers to these warning signs, ML systems can help save lives and reduce the burden on healthcare systems.
Challenges and Ethical Considerations
While the potential of machine learning in healthcare is boundless, it’s not without challenges. One major hurdle is the quality and accessibility of data. Machine learning models are only as good as the data they’re trained on, and issues like incomplete records, biased datasets, and data privacy concerns can limit their effectiveness. Ensuring that these algorithms are trained on diverse and representative datasets is crucial to avoid perpetuating health disparities. That said, you could argue effectively that the same limitations and risks exist under current healthcare regimes, run and operated by people.
Ethical considerations also come into play. Who is responsible if an ML algorithm makes an incorrect diagnosis? How do we ensure that patient data is used responsibly and transparently? These questions underscore the need for robust regulatory frameworks and ethical guidelines as machine learning becomes more integrated into healthcare.
The Future of Machine Learning in Healthcare
As machine learning continues to advance, its impact on healthcare will only grow. We’re already seeing the emergence of AI-powered virtual health assistants, wearable devices that monitor chronic conditions, and even robotic surgeons guided by ML algorithms. The possibilities are endless, and the potential to improve patient outcomes is profound.
But perhaps the most exciting aspect of machine learning in healthcare is its ability to democratize access to high-quality care. By automating routine tasks, reducing diagnostic errors, and enabling early intervention, ML has the power to make healthcare more efficient, affordable, and equitable for all.
Conclusion
Machine learning is not just transforming healthcare—it’s redefining what’s possible. From early diagnosis to personalized treatment and predictive analytics, this technology is empowering clinicians, improving patient outcomes, and saving lives. As we navigate the challenges and ethical considerations, one thing is clear: the future of healthcare is intelligent, data-driven, and full of promise. The question is no longer whether machine learning will revolutionize healthcare, but how quickly we can harness its potential to create a healthier world for everyone.
Written/published by Kevin Marshall with the help of AI models (AI Quantum Intelligence)