
Photo by ROMAN ODINTSOV
Imagine a world where machines are able to understand what you say and how you feel; where you can talk to a computer, and it’ll respond; and where technology can sift through the text and summarize it for you. Wait a minute. You don’t have to imagine anything — it’s a reality today, with the NLP adoption.
As a subfield of AI, natural language processing (NLP) has emerged as a breakthrough in technology, enabling computers to communicate using human language. Its market size was valued at $18.9 billion in 2023 and is expected to grow to $68 billion by 2028. Nothing’s surprising about this, regarding the diverse applications of NLP in the modern-day world, from chatbots to machine translation to document analysis.
In this post, we discuss the transformative impact of NLP on business, its use cases, and real-world examples per industry. We also briefly touch on the benefits of natural language processing, its challenges, and the future opportunities it brings to us.
NLP is a combination of linguistic, statistical, and machine learning (ML) techniques that allow the processing of massive amounts of data. This enables computers to grasp the nuances in human language, understand the context, and respond to it in a meaningful way. In other words, NLP algorithms aim to bridge human communication with AI.
But this wasn’t always the case. The infographic below covers the evolution of NLP over time until it reached today’s potential. The key driving factors for NLP adoption were improvements in computational power, advancements in AI and machine learning, and data availability. The latter occurred largely because of the cloud, which provided better scalability and lower costs for data storage and processing.

The Evolution of NLP
The evolution of NLP was also all about a transition from rule-based systems to ML algorithms, which can learn to “understand” the language. In a rule-based approach, an expert manually encodes each rule in NLP. That’s why these systems are more static and non-adaptable as compared to machine learning.
Exploring further the nature of NLP, we should mention its fundamental objectives to understand and interact with the human language. Accordingly, we distinguish between:
- Natural Language Understanding (NLU), which is concerned with the extraction of meaning. It helps to comprehend the intricacies and nuances of the written and spoken language, dealing with ambiguities and contextual variations. For instance, NLU comes in handy to differentiate between accents or understand slang.
- Natural Language Generation (NLG), which deals with producing human-like responses from data. Using statistical methods and language models to analyze big volumes of data, NLG helps to “respond” to user queries in a conversational manner. It also handles text summarization, machine translation, and content creation.
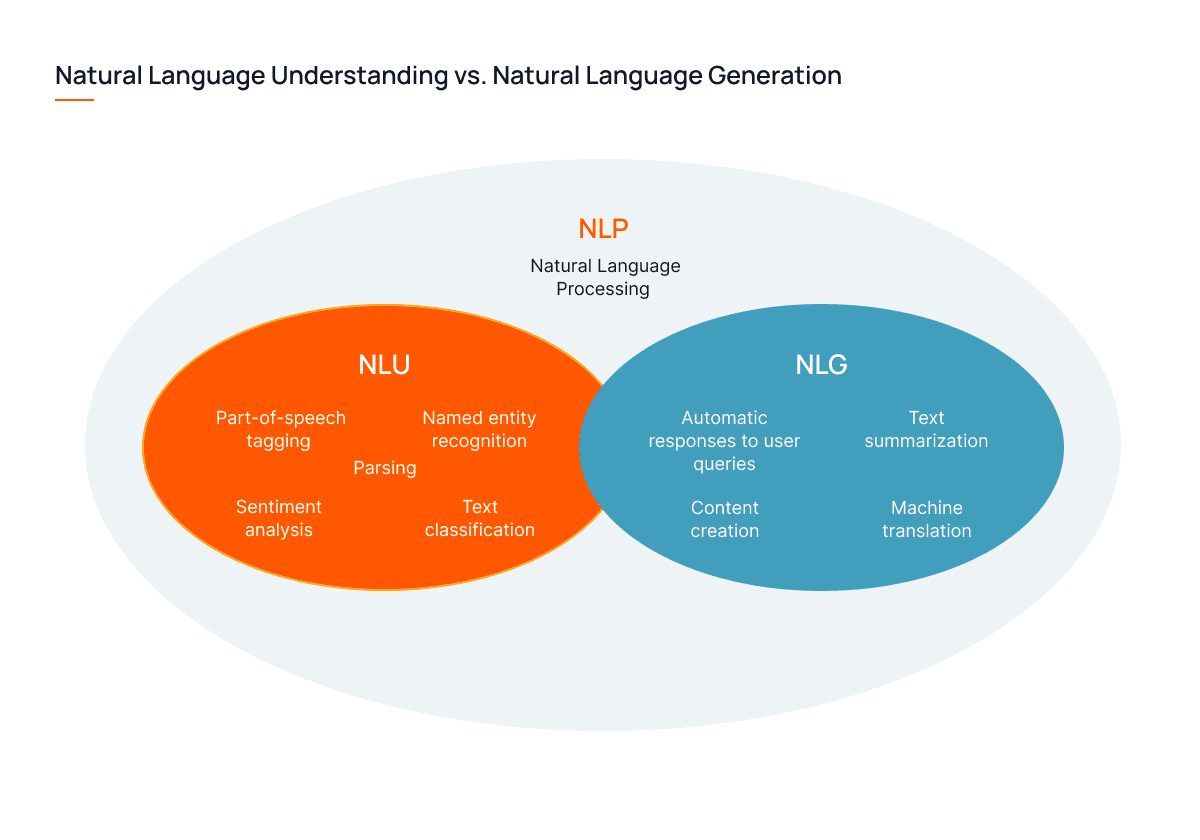
Natural Language Understanding vs. Natural Language Generation
Now we can outline how NLP works. Basically, there are 2 steps:
- Transforming the text into something a machine can understand
- Analyzing the text to actually understand the context and language and extract the meaning
At the same time, a lot is going on under the hood of a natural language processing system to enable the machine to perform these two actions. Let’s have a quick look at the key NLP components to understand how it works better:
- Tokenization: To break down a text into smaller units like words or phrases (tokens) to process them in smaller, manageable chunks
- Syntax analysis: To parse grammatical structures to properly understand syntactic relationships in sentences
- Part-of-speech tagging: To assign grammatical tags (e.g., noun, verb, etc.) to words in a sentence to add to the syntactic analysis
- Semantic analysis: To catch the meaning and context behind words, phrases, and sentences
- Sentiment analysis: To determine the sentiment or emotion expressed in the text like positive, negative, or neutral
- Named Entity Recognition (NER): To identify and categorize entities, i.e. names, organizations, locations, etc.
- Statistical and ML models: To process and analyze volumes of data. Supervised ML algorithms work best for tasks like text classification and sentiment analysis while unsupervised algorithms for clustering and topic modeling.
- Language models: To predict the probability of word sequences in a context. This technique is widely used in auto-completion and language generation tasks
- Language translation models: To convert text from one language to another. Advanced models, such as neural machine translation, can significantly add to the accuracy of translation.
- Language generation techniques: To generate human-like responses based on data or given context. This approach is used for chatbots, text summarization, etc.
The combination and integration of these components allow data scientists to build powerful NLP systems and contribute to better AI communication results.
Natural language processing is only gaining momentum across industries, and new applications appear every year. Below we review the most common use cases of NLP to discover more about your business potential of transforming communication with NLP.

Top Applications of NLP
Conversational AI and chatbots
Smart virtual assistants and chatbots are the first that comes to your mind when thinking about NLP. Today’s NLP conversational AI systems are sophisticated enough to engage in authentic and contextually appropriate dialogues with users.
Virtual assistants like Siri or Alexa are in our everyday use, handling minor tasks like setting reminders, making and receiving phone calls, and finding where to park. NLP-driven chatbots contribute to businesses by scaling support services and improving personalization.
Look at the Lyro chatbot below developed by Tidio. Unlike regular chatbots, Lyro doesn’t require any training from support agents — the company activates it and starts responding to users’ queries right away.

Image from Tidio
Machine Translation
Machine translation is the second most prominent use case of NLP. Students, language translators, tourists, and many others can’t imagine their lives without Google Translate today. And although machine translation existed long before NLP, it has brought it to a new level by:
- Adding more accuracy and fluency with the use of transformers
- Promoting and facilitating real-time language translation
- Making possible context-aware translation, which left traditional word-for-word translation methods in the past
- Helping with content localization to consider cultural preferences and local dialects
To make it more illustrative, here’s DeepL, a less known competitor to Google Translate. The tool supports translation into 26 languages to help users break down language barriers. It also has app integration and a website translation widget.

Image from Deepl
Document Management
NLP also has unique speech-to-text capabilities that help to improve the accuracy and efficiency of documentation. Leaving out simplistic use cases like dictating text instead of typing it, we can also speak about the following:
- Text summarization: Automatic summaries delivered by AI are useful when one needs to digest large amounts of information quickly. NLP doesn’t simply summarize lengthy texts — keyword extraction and sentence ranking allow NLP to summarize a text in a coherent manner by capturing key points.
- Information extraction: Among other approaches in NLP, NER is especially efficient for automated information retrieval and knowledge discovery. This significantly saves time and effort for a researcher not to skim through a vast amount of information.
- Text classification: When it comes to large volumes of textual data, NLP can help to categorize it. While getting the data more organized, the company also benefits from improved information accessibility.
Content Generation
Due to its ability to catch the essence of events and data, NLP can generate content based on the given information. Probably everyone has already heard of ChatGPT and how it creates unique, meaningful content with the right prompts. Models like this can make the lives of content creators easier by helping them write product instructions, social media posts, articles, emails, and others.
Consider a less mainstream example of an AI content creator tool than GPT. OwlyWriter AI can save hours of marketer’s work when it comes to SMM. From creating captions for posts to generating content ideas to writing posts, the tool helps social media professionals beat writer’s block and work more efficiently.

Image from Hootsuite
Speech Recognition
Another great application of NLP is speech recognition, which allows machines to translate spoken language into written text. Again, voice assistants like Siri or Google Assistant are the most illuminating examples in this case.
There are still lots of other use cases for speech recognition like transcription services or voice-controlled devices. Remember about the feature that allows drivers to control cars safely hands-free. Also, smart home devices are all developed based on NLP.
Sentiment Analysis
Sentiment analysis, as one of the NLP techniques, works best to analyze customer reviews and social media sentiments to get public opinions about the product or service or follow trends.
For instance, NLP can help businesses analyze customer feedback about the recent product launch to make more informed decisions for customer satisfaction. It also powers up apps for social media monitoring, like Brandwatch. These monitor social network content for companies to know public opinions and feelings toward brands, track trends, and manage online reputation.

Image by Brandwatch
Search Engine Optimization
Search engines like Google use NLP to improve the accuracy of their search results. This approach helps to understand the user intent behind the query better and match it with the most relevant search results.
Spam Filtering
One more area that NLP has revolutionized includes spam filtering. And here we speak not only about email but other applications too. For example, YouTube uses NLP to filter spam data in the comment section of its videos. It uses a tool called TubeSpam, which was trained using the Naïve Bayes classifier to filter out spam.
The list of NLP applications is much longer. We discussed the biggest use cases but left out smaller ones like autocorrect and autocomplete features, fraud detection, etc. To make our research fuller, let’s speak about real-life examples of how NLP transforms industries.
Although NLP is successfully implemented across various industries, its biggest market share is in tech, healthcare, retail, financial services, insurance, and marketing. See about each of these in detail.

Global NLP Market Share by Industry
Customer Service
NLP-driven chatbots and virtual assistants have altered customer service once and forever. Now, customers can get 24/7 support while agents benefit from reduced workload. Erica — the chatbot created by Bank of America — provides financial support and guidance to clients and helps to navigate online banking. NLP enables Erica to learn users’ preferences and needs and provide personalized recommendations.
Let’s look at specific examples of the use of NLP in customer service:
- NLP-based voice assistants to understand the user’s request and direct it to the right human agent
- Automated chatbots for simple tasks like answering questions, checking information, scheduling appointments, etc.
- Use of virtual assistants for hands-free interactions with devices and services
- Customer feedback analysis and sentiment analysis — for example, a chatbot can start with an apology when dealing with a frustrated customer
E-commerce and Retail
Most reputable e-commerce websites today like Amazon, eBay, or Walmart use NLP-powered semantic search, which improves product visibility and search experience. In contrast to matching keywords, semantic search is more intuitive and aims to understand the user’s intent behind the query.
Next to semantic search, NLP has other applications in retail:
- Customer sentiment analysis to understand more about brand loyalty and ultimately strengthen the brand
- Conversational commerce with voice assistants
- Personalized product recommendations
Education
In the field of education, NLP has the most creative use cases. A great example includes Coursera’s course recommendation system, which helps users find the best courses based on their interests. Think also about all-favorite Grammarly, an NLP-based solution that makes your writing clear and error-free.
Other examples of NLP in education include but are not limited to:
- Intelligent tutoring systems
- Exam question generation based on textbooks or other training materials
- Automated grading and feedback analysis
- Plagiarism detection software
- Adaptive learning and personalized instruction and feedback to students
Finances and Banking
Have you ever received a call from the bank asking about suspicious activity on your account? These calls are usually triggered by NLP. Fraud detection is one of the biggest applications of NLP in finances. At one time, Mastercard Decision Intelligence, which was specifically developed to indicate fraudulent activity, helped the company to cut fraud by 50%. Check the solution potential by yourself:
Alt tag: Mastercard Decision Intelligence
Two other ways of how NLP is employed in the financial sector include:
- Sentiment analysis on various text data like financial reports, social media posts, and news articles to predict stock prices and market fluctuations and, thus, help traders and investors make more informed decisions
- Data extraction from financial reports and documents as well as summarization of financial news for quick updates
Healthcare
NLP technology is helpful to medical providers to summarize and categorize clinical notes and patient information. This way, they can access data faster and keep documentation organized. Electronic health records became possible mostly thanks to natural language processing.
Also, NLP can assist in transcription allowing physicians to dictate notes and minimize manual data entry. Clinical NLP systems can help with diagnoses, treatment plan composition, and personalized therapy recommendations. For example, Merative L.P. uses NLP algorithms for the development of cancer treatment plans for its patients.
Insurance
Like in finances, NLP in insurance is employed to identify fraudulent claims. By analyzing different types of data like customer profiles, communication, and social networks, NLP detects indicators of fraud and sends these claims for further inspection. The Turkish insurance company improved ROI by 210% after they switched to the ML-based fraud detection system.
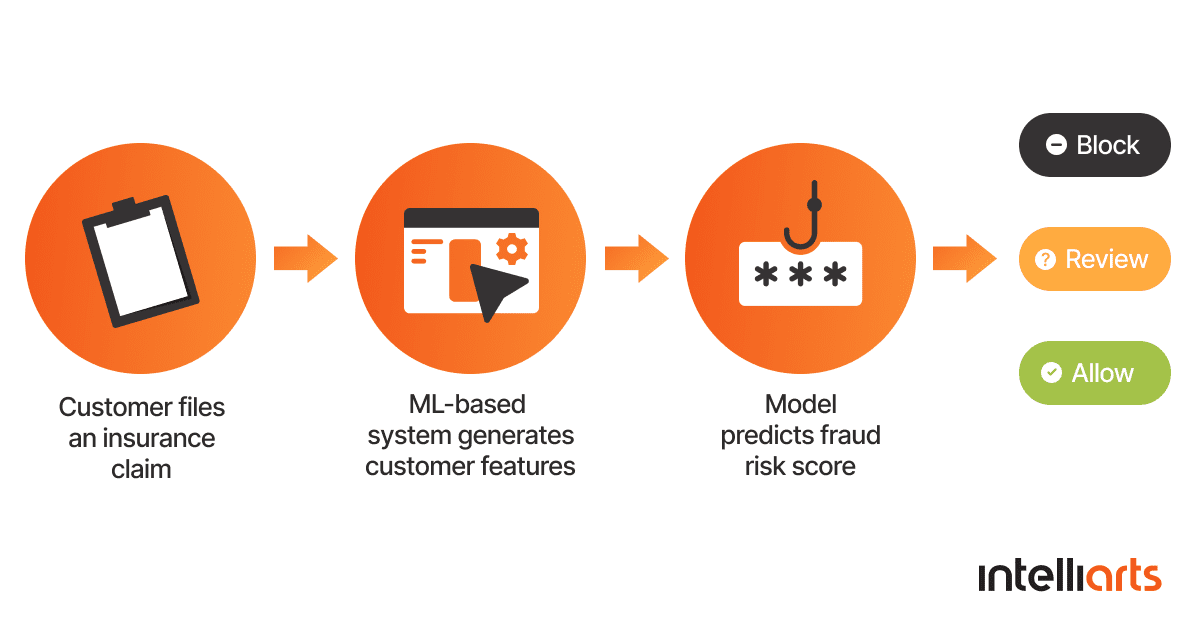
How an ML fraud detection system works
Insurance businesses can also benefit from NLP by monitoring industry trends with the help of text mining and market intelligence. This way, companies get insights into how the competitors are doing and make more data-driven decisions.
Law
In the legal sector, NLP is the most helpful when it comes to working with documents. Legal professionals can use this technology in contract review and analysis, text summarization, case outcome analysis, etc. NLP algorithms help attorneys and lawyers scan through lots of legal texts to find specific dates, terms, or clauses.
Luminance uses NLP to increase the efficiency of due diligence and contract review. In contrast to more generalist GPT, the model was trained on 150+ million legal documents and verified by industry experts. The company promises users up to 90% time savings through automated contract processing.
Apart from this, legal professionals apply NLP in regulatory compliance monitoring, surveillance transcript analysis, and legal research.
Manufacturing and Supply Chain
As everywhere else, NLP in manufacturing and supply chain works best to keep data organized and streamline communication. For example, it can help to analyze and sift through volumes of shipment documents and solve logistical challenges.
Chatbots can be useful to respond to customer or vendor queries faster. Tesla incorporated chatbots to provide exceptional customer experiences a long time ago. These schedule test drives and answer simple questions about Tesla cars.
By integrating chatbots with the manufacturer’s ERP or other legacy systems, chatbots can also help to keep information in one place and improve collaboration among departments.
Marketing
As mentioned, sentiment analysis is widely used in marketing to understand customer opinions about brands. This helps to suggest personalized products or services to customers and power up decision-making. For instance, McDonald’s uses NLP to monitor customer complaints on social media and train employees to respond to these complaints correctly.
With the help of NER, NLP is also leveraged to identify trending topics and customer insights to use them further in sales materials or product design improvements.
Recruitment
In recruitment, NLP is used for job candidate screening to improve accuracy and speed. For example, a B2B job sourcing platform developed by Intelliarts can match candidate profiles on job search sites and social media sites like LinkedIn with position descriptions. What’s more, the solution sticks to the Diversity, Equity, and Inclusion (DEI) principles. On the way out, the customer gets streamlined candidate sourcing but with DEI requirements as intended.

B2B Job Sourcing Platform
Despite the increasing popularity of NLP in industries and its advancement, some challenges still exist on its way to integration into existing systems. Here are both the challenges and potential solutions to them:
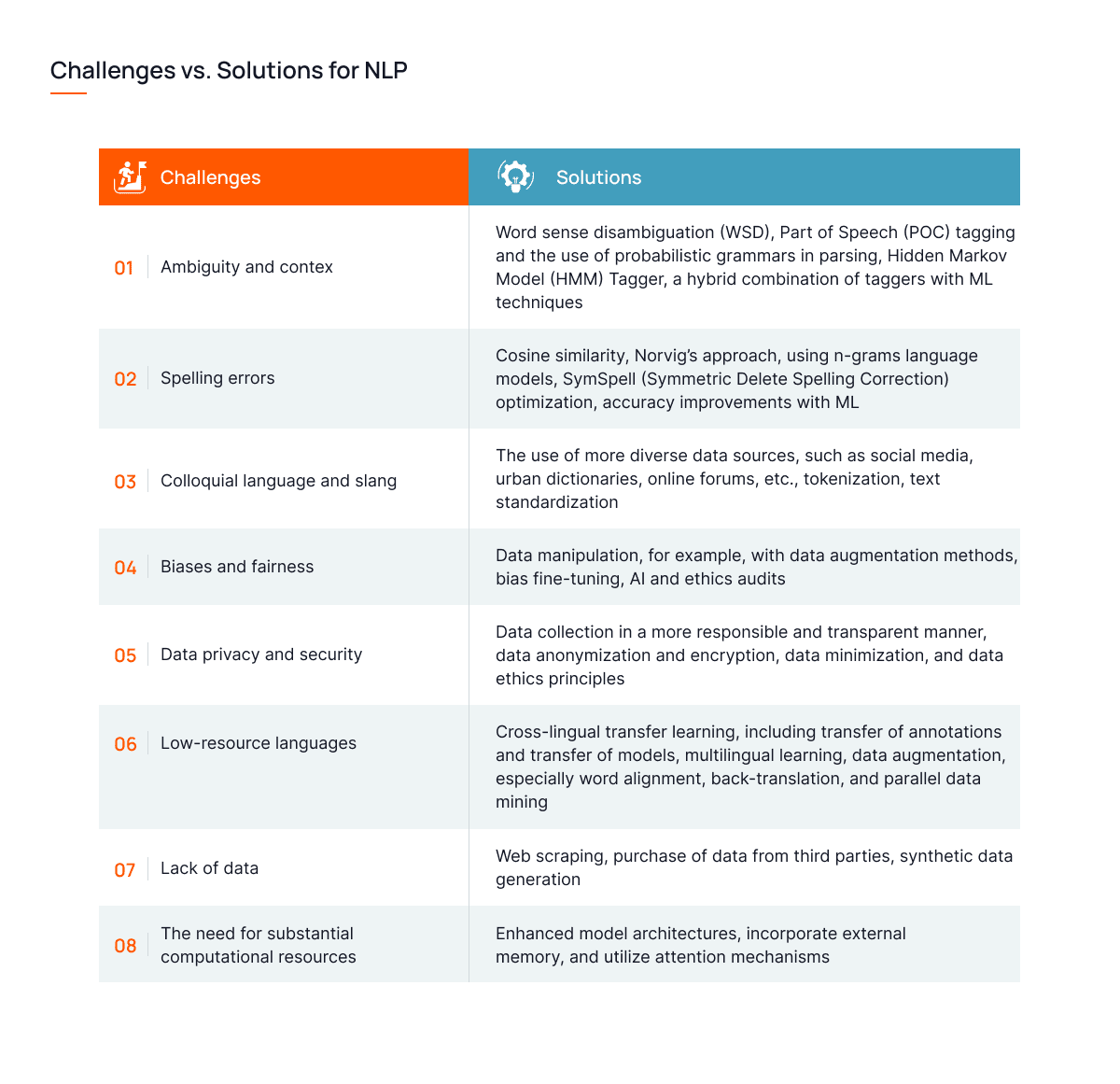
Challenges vs. Solutions for NLP
NLP continues to develop, with new solutions emerging to tackle the challenges above. At the same time, new applications and trends appear in NLP research. Let’s see the latest developments of NLP and how these could revolutionize human-AI interactions even further:
- Pre-training and transfer learning: Pre-trained models like GPT-3 or T5 are one of the most important advancements in NLP today. And this trend stays for sure due to its efficient results, as well as the opportunity of transfer learning to adapt knowledge learned from one task to other tasks and domains.
- Multimodal NLP: NLP finally goes beyond text, and researchers try its capabilities in speech, videos, and images. Multimodality finds its applications in different areas, from video captioning to autonomous vehicles to more accurate sentiment analysis.
- Conversational AI: Multimodality of NLP also displays itself in advances of conversational AI, which aims to make human-AI interaction more natural and intuitive. Voice assistants for smart homes are probably of the most interest to researchers now.
- Multilingual NLP: Multilingual and cross-lingual NLP interest researchers because of its opportunities to enhance global communication, increase information access and cultural diversity.
- Explainable and trustworthy AI: The demand for explainable and trustworthy AI refers to empowering user confidence, accountability, and responsibility in NLP. This is especially relevant for sensitive domains like healthcare, education, and law.
- Ethical and responsible AI: Researchers also aim to address biases, fairness, and ethical concerns in NLP to create more responsible AI applications. A great example here is deepfake detection to identify and flag AI-manipulated video and audio information.

Areas of Ongoing Research in NLP
The concept of NLP has revolutionized human-machine interactions, reshaping how information is accessed and communication occurs. Through the integration of AI with deep learning, computers gained the ability to read texts, interpret speech, analyze conversations, determine sentiments, and many more, proving the power of NLP in extracting valuable insights from data.
We see endless possibilities of NLP nowadays, ranging from chatbots and virtual assistants to sentiment analysis to language translation. These have already transformed many industries and elevated user experiences. But ongoing research and development in NLP promise an even brighter future marked by more advancements and trends. This has the potential to make communication more seamless and inclusive than ever before.
Olena Zherebetska is a content writer at Intelliarts, writing about the latest news and innovations in data science and ML. She has 7 years of writing experience and loves to go deeper when researching tech topics.