Your Company Needs Small Language Models
Image generated by Stable DiffusionWhen specialized models outperform general-purpose models“Bigger is always better” — this principle is deeply rooted in the AI world. Every month, larger models are created, with more and more parameters. Companies are even building $10 billion AI data centers for them. But is it the only direction to go?At NeurIPS 2024, Ilya Sutskever, one of OpenAI’s co-founders, shared an idea: “Pre-training as we know it will unquestionably end”. It seems the era of scaling is coming to a close, which means it’s time to focus on improving current approaches and algorithms.One of the most promising areas is the use of small language models (SLMs) with up to 10B parameters. This approach is really starting to take off in the industry. For example, Clem Delangue, CEO of Hugging Face, predicts that up to 99% of use cases could be addressed using SLMs. A similar trend is evident in the latest requests for startups by YC:Giant generic models with a lot of parameters are very impressive. But they are also very costly and often come with latency and privacy challenges.In my last article “You don’t need hosted LLMs, do you?”, I wondered if you need self-hosted models. Now I take it a step further and ask the question: do you need LLMs at all?“Short” summary of the article.In this article, I’ll discuss why small models may be the solution your business needs. We’ll talk about how they can reduce costs, improve accuracy, and maintain control of your data. And of course, we’ll have an honest discussion about their limitations.Cost EfficiencyThe economics of LLMs is probably one of the most painful topics for businesses. However, the issue is much broader: it includes the need for expensive hardware, infrastructure costs, energy costs and environmental consequences.Yes, large language models are impressive in their capabilities, but they are also very expensive to maintain. You may have already noticed how subscription prices for LLMs-based applications have risen? For example, OpenAI’s recent announcement of a $200/month Pro plan is a signal that costs are rising. And it’s likely that competitors will also move up to these price levels.$200 for Pro planThe Moxie robot story is a good example of this statement. Embodied created a great companion robot for kids for $800 that used the OpenAI API. Despite the success of the product (kids were sending 500–1000 messages a day!), the company is shutting down due to the high operational costs of the API. Now thousands of robots will become useless and kids will lose their friend.One approach is to fine-tune a specialized Small Language Model for your specific domain. Of course, it will not solve “all the problems of the world”, but it will perfectly cope with the task it is assigned to. For example, analyzing client documentation or generating specific reports. At the same time, SLMs will be more economical to maintain, consume fewer resources, require less data, and can run on much more modest hardware (up to a smartphone).Comparison of utilization of models with different number of parameters. Source1, source2, source3, source4.And finally, let’s not forget about the environment. In the article Carbon Emissions and Large Neural Network Training, I found some interesting statistic that amazed me: training GPT-3 with 175 billion parameters consumed as much electricity as the average American home consumes in 120 years. It also produced 502 tons of CO₂, which is comparable to the annual operation of more than a hundred gasoline cars. And that’s not counting inferential costs. By comparison, deploying a smaller model like the 7B would require 5% of the consumption of a larger model. And what about the latest o3 release?Model o3 CO₂ production. Source.????Hint: don’t chase the hype. Before tackling the task, calculate the costs of using APIs or your own servers. Think about scaling of such a system and how justified the use of LLMs is.Performance on Specialized TasksNow that we’ve covered the economics, let’s talk about quality. Naturally, very few people would want to compromise on solution accuracy just to save costs. But even here, SLMs have something to offer.In-domain Moderation Performance. Comparing the performance of SLMs versus LLMs on accuracy, recall, and precision for in-domain content moderation performance. Best performing SLMs outperform LLMs on accuracy and recall across all subreddits, while LLMs outperform SLMs on precision. Source.Many studies show that for highly specialized tasks, small models can not only compete with large LLMs, but often outperform them. Let’s look at a few illustrative examples:Medicine: The Diabetica-7B model (based on the Qwen2–7B) achieved 87.2% accuracy on diabetes-related tests, while GPT-4 showed 79.17% and Claude-3.5–80.13%. Despite this, Diabetica-7B is dozens of times smaller than GPT-4 and can run locally on a consumer GPU.Legal Sector: An SLM with just 0.2B parameters achieves 77.2% accuracy in contract an

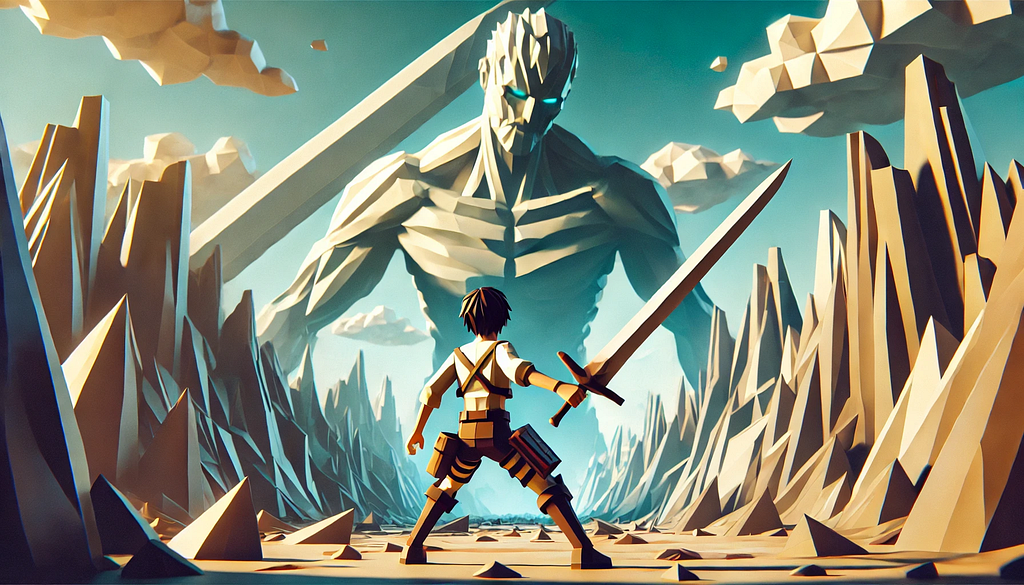
When specialized models outperform general-purpose models
“Bigger is always better” — this principle is deeply rooted in the AI world. Every month, larger models are created, with more and more parameters. Companies are even building $10 billion AI data centers for them. But is it the only direction to go?
At NeurIPS 2024, Ilya Sutskever, one of OpenAI’s co-founders, shared an idea: “Pre-training as we know it will unquestionably end”. It seems the era of scaling is coming to a close, which means it’s time to focus on improving current approaches and algorithms.
One of the most promising areas is the use of small language models (SLMs) with up to 10B parameters. This approach is really starting to take off in the industry. For example, Clem Delangue, CEO of Hugging Face, predicts that up to 99% of use cases could be addressed using SLMs. A similar trend is evident in the latest requests for startups by YC:
Giant generic models with a lot of parameters are very impressive. But they are also very costly and often come with latency and privacy challenges.
In my last article “You don’t need hosted LLMs, do you?”, I wondered if you need self-hosted models. Now I take it a step further and ask the question: do you need LLMs at all?
In this article, I’ll discuss why small models may be the solution your business needs. We’ll talk about how they can reduce costs, improve accuracy, and maintain control of your data. And of course, we’ll have an honest discussion about their limitations.
Cost Efficiency
The economics of LLMs is probably one of the most painful topics for businesses. However, the issue is much broader: it includes the need for expensive hardware, infrastructure costs, energy costs and environmental consequences.
Yes, large language models are impressive in their capabilities, but they are also very expensive to maintain. You may have already noticed how subscription prices for LLMs-based applications have risen? For example, OpenAI’s recent announcement of a $200/month Pro plan is a signal that costs are rising. And it’s likely that competitors will also move up to these price levels.
The Moxie robot story is a good example of this statement. Embodied created a great companion robot for kids for $800 that used the OpenAI API. Despite the success of the product (kids were sending 500–1000 messages a day!), the company is shutting down due to the high operational costs of the API. Now thousands of robots will become useless and kids will lose their friend.
One approach is to fine-tune a specialized Small Language Model for your specific domain. Of course, it will not solve “all the problems of the world”, but it will perfectly cope with the task it is assigned to. For example, analyzing client documentation or generating specific reports. At the same time, SLMs will be more economical to maintain, consume fewer resources, require less data, and can run on much more modest hardware (up to a smartphone).
And finally, let’s not forget about the environment. In the article Carbon Emissions and Large Neural Network Training, I found some interesting statistic that amazed me: training GPT-3 with 175 billion parameters consumed as much electricity as the average American home consumes in 120 years. It also produced 502 tons of CO₂, which is comparable to the annual operation of more than a hundred gasoline cars. And that’s not counting inferential costs. By comparison, deploying a smaller model like the 7B would require 5% of the consumption of a larger model. And what about the latest o3 release?
????Hint: don’t chase the hype. Before tackling the task, calculate the costs of using APIs or your own servers. Think about scaling of such a system and how justified the use of LLMs is.
Performance on Specialized Tasks
Now that we’ve covered the economics, let’s talk about quality. Naturally, very few people would want to compromise on solution accuracy just to save costs. But even here, SLMs have something to offer.
Many studies show that for highly specialized tasks, small models can not only compete with large LLMs, but often outperform them. Let’s look at a few illustrative examples:
- Medicine: The Diabetica-7B model (based on the Qwen2–7B) achieved 87.2% accuracy on diabetes-related tests, while GPT-4 showed 79.17% and Claude-3.5–80.13%. Despite this, Diabetica-7B is dozens of times smaller than GPT-4 and can run locally on a consumer GPU.
- Legal Sector: An SLM with just 0.2B parameters achieves 77.2% accuracy in contract analysis (GPT-4 — about 82.4%). Moreover, for tasks like identifying “unfair” terms in user agreements, the SLM even outperforms GPT-3.5 and GPT-4 on the F1 metric.
- Mathematical Tasks: Research by Google DeepMind shows that training a small model, Gemma2–9B, on data generated by another small model yields better results than training on data from the larger Gemma2–27B. Smaller models tend to focus better on specifics without the tendency to “trying to shine with all the knowledge”, which is often a trait of larger models.
- Content Moderation: LLaMA 3.1 8B outperformed GPT-3.5 in accuracy (by 11.5%) and recall (by 25.7%) when moderating content across 15 popular subreddits. This was achieved even with 4-bit quantization, which further reduces the model’s size.

I’ll go a step further and share that even classic NLP approaches often work surprisingly well. Let me share a personal case: I’m working on a product for psychological support where we process over a thousand messages from users every day. They can write in a chat and get a response. Each message is first classified into one of four categories:
- SUPPORT — A question about how the app works; we respond using the documentation.
- GRATITUDE — The user thanks the bot; we simply send a “like.”
- TRY_TO_HACK — The user requests something unrelated to the app’s purpose (e.g., “Write a function in Python”).
- OTHER— All other messages, which we process further.
Previously, I used GPT-3.5-turbo for classification and later switched to GPT-4o mini, spending a lot of time changing the prompt. However, I still encountered errors. So, I decided to try a classic approach: TF-IDF + a simple classifier. Training took less than a minute, and the Macro F1 score increased to 0.95 (compared to 0.92 for GPT-4o mini). The model size is just 76 MB, and when applied to 2 million processed messages (our actual data), the cost savings were significant: the GPT-based solution would have cost about $500, while the classic approach cost almost nothing.
And there are several such “small” and simple tasks in our product. I believe you might find the same in your company. Of course, large models are great for a quick start, especially when there’s no labeled data and requirements are changing. But for well-defined, stable tasks where accuracy and minimal costs are key, specialized and simple models (including classic methods) can often be a more effective solution.
????Hint: use LLMs for prototyping, and then, once the task becomes clear and stable, switch to smaller, cheaper, and more accurate models. This hybrid approach helps maintain high quality, significantly reduce costs, and avoid the redundancy of general-purpose models.
Security, Privacy and Regulatory
Using LLMs through APIs, you’re handing over sensitive data to external providers, increasing the risk of leaks and complicating compliance with strict regulations like HIPAA, GDPR, and CCPA. OpenAI’s recent announcement about plans to introduce advertising only highlights these risks. Your company not only loses full control over its data but also becomes dependent on third-party SLAs.
Certainly, it’s possible to run a LLM locally, but the cost of deployment and scaling (hundreds of gigabytes of memory, multiple GPUs) often exceeds reasonable economic limits and makes it difficult to quickly adapt to new regulatory requirements. And you can forget about launching it on low-end hardware.
And this is where the “small guys” come back into play:
1. Simplified Audits
The smaller size of SLMs lowers the barrier for conducting audits, verification, and customization to meet specific regulations. It’s easier to understand how the model processes data, implement your own encryption or logging, and show auditors that information never leaves a trusted environment. As the founder of a healthcare company, I know how challenging and crucial this task can be.
2. Running on Isolated and low-end hardware
LLMs are difficult to efficiently “deploy” in an isolated network segment or on a smartphone. SLMs, however, with their lower computational requirements, can operate almost anywhere: from a local server in a private network to a doctor’s or inspector’s device. According to IDC forecasts, by 2028, over 900 million smartphones will be capable of running generative AI models locally.
3. New Regulations Updates and Adaptation
Regulations and laws change frequently — compact models can be fine-tuned or adjusted in hours rather than days. This enables a quick response to new requirements without the need for large-scale infrastructure upgrades, which are typical for big LLMs.
4. Distributed Security Architecture
Unlike the monolithic architecture of LLMs, where all security components are “baked” into one large model, SLMs enable the creation of a distributed security system. Each component:
- Specializes in a specific task.
- Can be independently updated and tested.
- Scales separately from the others.
For example, a medical application could use a cascade of three models:
- Privacy Guardian (2B) — masks personal data.
- Medical Validator (3B) — ensures medical accuracy.
- Compliance Checker (1B) — monitors HIPAA compliance.
Smaller models are easier to verify and update, making the overall architecture more flexible and reliable.
????Hint: consider using SLMs if you operate in a heavily regulated field. Pay close attention to data transfer policies and the frequency of changes in the regulatory landscape. I recommend use SLMs if your professional domain is in healthcare, finance, or law.
AI Agents: The Perfect Use Case
Remember the old Unix philosophy, “Do one thing and do it well”? It seems we’re returning to this principle, now in the context of AI.
Ilya Sutskever’s recent statement at NeurIPS that “Pre-training as we know it will unquestionably end” and that the next generation of models will be “agentic in real ways” only confirms this trend. Y Combinator goes even further, predicting that AI agents could create a market 10 times larger than SaaS.
For example, already 12% of enterprise solutions use agent-based architecture. Moreover, analysts predict that agents will be the next wave of AI-transformation that can affect not only the $400-billion software market, but also the $10-trillion U.S. services economy.
And SMLs are ideal candidates for this role. Perhaps one model is quite limited, but a swarm of such models — can solve complex tasks piece by piece. Faster, higher quality and cheaper.
Let’s take a concrete example: imagine you are building a system to analyze financial documents. Instead of using one large model, you can break the task into several specialized agents:
And this approach is not only more cost-effective but also more reliable: each agent focuses on what it does best. Cheaper. Faster. Better. Yes, I’m repeating it again.
To back this up, let me name a few companies:
- H Company raised $100M in a seed round to develop a multi-agent system based on SLMs (2–3B parameters). Their agent Runner H (3B) achieves 67% task completion success compared to Anthropic’s Computer Use at 52%, all with significantly lower costs.
- Liquid AI recently secured $250M in funding, focusing on building efficient enterprise models. Their model (1.3B parameters) has outperformed all existing models of similar size. Meanwhile, their LFM-3B delivers performance on par with 7B and even 13B models while requiring less memory.
- Cohere launched Command R7B, a specialized model for RAG applications that can even run on a CPU. The model supports 23 languages and integrates with external tools, showing best-in-class results for reasoning and question-answering tasks.
- YOUR COMPANY NAME could also join this list. I’m not just saying that — in Reforma Health, the company I’m working on, is developing specialized SLMs for various medical domains. This decision was driven by the need to comply with HIPAA requirements and the specifics of medical information processing. Our experience shows that highly specialized SLMs can be a significant competitive advantage, especially in regulated domains.
These examples highlight the following:
- Investors believe in the future of specialized small models.
- Enterprise clients are willing to pay for efficient solutions that don’t require sending data to external providers.
- The market is shifting towards “smart” specialized agents instead of relying on “universal” large models.
????Hint: start by identifying repetitive tasks in your project. These are the best candidates for developing specialized SLM agents. This approach will help you avoid overpaying for the excessive power of LLMs and achieve greater control over the process.
Potential Limitations of SLMs Compared to LLMs
Although I’ve spent this entire article praising small models, it’s fair to point out their limitations as well.
1. Limited Task Flexibility
The most significant limitation of SLMs is their narrow specialization. Unlike LLMs, which can handle a wide range of tasks, SLMs succeed only in the specific tasks for which they have been trained. For example, in medicine, Diabetica-7B outperformed LLMs in diabetes-related tests, but other medical disciplines required additional fine-tuning or a new architecture.
2. Context Window Limitations
Unlike large models that reach up to 1M tokens (Gemini 2.0), SLMs have shorter contexts. Even though recent advances in small LLaMA 3.2 models (3B, 1B) having a context length of 128k tokens, the effective context length is often not as claimed: models often lose the “connection” between the beginning and the end of the text. For example, SLMs cannot efficiently process voluminous medical histories of patients over several years or large legal documents.
3. Emergence Capabilities Gap
Many “emergent abilities” only appear when a model reaches a certain size threshold. SLMs typically don’t hit the parameter levels required for advanced logical reasoning or deep contextual understanding. A study by Google Research demonstrates this with math word problems: while small models struggle with basic arithmetic, larger models suddenly demonstrate complex mathematical reasoning skills.
However, recent research by Hugging Face shows that test-time compute scaling can partially bridge this gap. Using strategies like iterative self-refinement or employing a reward model, small models can “think longer” on complex problems. For example, with extended generation time, small models (1B and 3B) outperformed their larger counterparts (8B and 70B) on the MATH-500 benchmark.
????Hint: If you work in an environment where tasks change weekly, require analyzing large documents, or involve solving complex logical problems, larger LLMs are often more reliable and versatile.
Closing thoughts
As with choosing between OpenAI and self-hosted LLMs in my previous article, there is no one-size-fits-all solution here. If your task involves constant changes, lacks precise specialization, or requires rapid prototyping, LLMs will offer an easy start.
However, over time, as your goal become more clearer, moving to compact, specialized SLM agents can significantly reduce costs, improve accuracy, and simplify compliance with regulatory requirements.
SLMs aren’t a paradigm shift for the sake of trends but a pragmatic approach that allows you to solve specific problems more accurately and cost-effectively without overpaying for unnecessary functionality. You don’t need to completely abandon LLMs — you can gradually replace only some components with SLMs or even classic NLP methods. It all depends on your metrics, budget, and the nature of your task.
A good example of this is IBM, which employs a multimodel strategy, combining smaller models for different tasks. As they point out:
Bigger is not always better, as specialized models outperform general-purpose models with lower infrastructure requirements.
In the end, the key to success is to adapt. Start with a large model, evaluate where it performs best, and then optimize your architecture to avoid overpaying for unnecessary capabilities and compromising data privacy. This approach allows you to combine the best of both worlds: the flexibility and versatility of LLMs during the initial stages, and the precise, cost-effective performance of SLMs for a mature product.
If you have any questions or suggestions, feel free to connect on LinkedIn.
Disclaimer: The information in the article is current as of December 2024, but please be aware that changes may occur thereafter.
Unless otherwise noted, all images are by the author.
Your Company Needs Small Language Models was originally published in Towards Data Science on Medium, where people are continuing the conversation by highlighting and responding to this story.